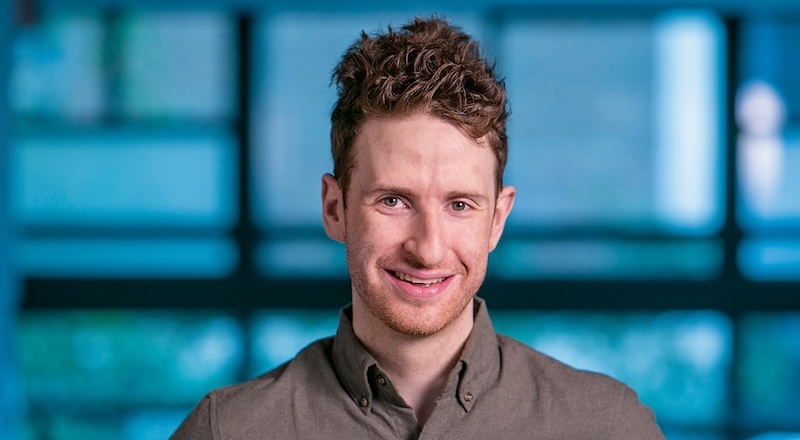
In North Carolina, where Jacob Gardner, Assistant Professor in Computer and Information Science, grew up, hurricanes arrive like unwelcome relatives — Fran, Matthew, Florence. In high school, Gardner interned for a company that attempted to predict hurricanes. “That was my first exposure to machine learning,” he recalls.
Machine learning, a form of artificial intelligence (AI), works by predicting the future using vast amounts of data from the past. As Gardner puts it, “We train models that take the data — examples we’ve seen — and we try to generalize new examples we haven’t seen.”
Today, Gardner applies machine learning not to weather prediction, but to scientific research. Rather than predict the movement of hurricanes, he develops tools that will allow scientists to supercharge fields like drug discovery. “I want to build the AI equivalents of the electron microscope,” he says. “Tools that help scientists do what they’re already doing, just faster, more effectively and with new insight.”
I want to build the AI equivalents of the electron microscope. Tools that help scientists do what they’re already doing, just faster, more effectively and with new insight.
Jacob Gardner, Assistant Professor in Computer and Information Science
Growing up, Gardner found himself fascinated by the intersection of computer science, chemistry and biology. Although his own talents lent themselves more to computing, he never lost sight of his interest in other fields. “I’ve always been excited about applications of computer science and how we can use computing to impact the physical world around us,” he says.
That interest drew him to Penn Engineering, where in 2022 he received a CAREER Award from the National Science Foundation to support his research on applying AI to science. Previously, at Uber, where Gardner worked as a research scientist after earning his Ph.D., he developed methods to optimize the choices machine learning algorithms make.
Given the collaborative nature of research at Penn Engineering, Gardner can now employ those techniques in projects with colleagues at the Perelman School of Medicine. “If you want to go from the conception of a drug using AI technology through to clinical trials,” Gardner points out, “Penn actually does clinical trials in house — talk about a collaborative environment.”
Ultimately, Gardner hopes to create the tools necessary to run a fully automated lab, which could discover the next generation of therapeutics for drug-resistant bacteria and diseases like cancer. “The dream is a ‘self-driving lab,’” Gardner says. “You could automate at least the first part of drug discovery, which is identifying interesting molecules.”
In his vision, such a lab would use AI to generate molecular structures for a particular class of therapeutics. Robots would then synthesize the compounds and test the molecules against threats like bacteria, viruses or cancer cells. The system would then ingest the results of the experiments. “Either it works well,” he says, “in which case your model predicted a great new candidate, or you’ve learned something, and you can update the model.”
The dream is a ‘self-driving lab.’ You could automate at least the first part of drug discovery, which is identifying interesting molecules.
Jacob Gardner, Assistant Professor in CIS
Ultimately, Gardner’s interest in optimization extends far beyond any specific domain. “I’m really motivated by challenging, iterative design problems,” he says, meaning problems to which AI can be applied that yield a tangible product in the real world, and that require repeated trial and error to get right. “Maybe it’s a drug, maybe it’s the deployment of solar panels on a roof.”
At the moment, AI tools are less skilled at evaluating their own efforts, especially in the areas that interest Gardner, like molecular design. “If I ask an AI to generate molecules or peptides,” Gardner says, “they often look like things we could at least manufacture in a lab.” But testing the molecule’s properties is something else. “How stable they are at different temperatures, how strongly they bind to targets of interest that might be useful for treating some disease — incorporating that iterative feedback into the generative process is really challenging.”
In addition to conducting research, Gardner also teaches the lecture course CIS 5200: Machine Learning, and is revising his seminar course on optimization to focus more heavily on applying AI to other scientific fields.
As a mentor, Gardner welcomes both graduate and undergraduate students to his research group, and encourages them to take on projects that excite them. “My philosophy with my graduate students is, they’re probably most productive when they’re working on things they’re excited about, so whatever they’re excited about is where we go.”
Since his lab focuses on applying AI to science, the students he works with can research everything from theoretical optimization problems to developing tools that could be applied in any number of fields. “One of the nice things about working in AI,” Gardner says, “is that the methods we work on are often quite general, and they generalize from domain to domain — that’s almost the power of AI.”
To learn more about Jacob Gardner and his research, please visit the Gardner Lab’s website. The Gardner Lab is currently recruiting graduate students for Fall 2025.